2024 Keynote Speakers
Prof. Stefania Montani
Università del Piemonte Orientale, Alessandria, Italy
Speech Title: From Process Traces to Process Models in A Knowledge-Intensive Way: Experiences In The Medical Domain
Stefania Montani received her Ph.D. in Bioengineering and Medical Informatics from the University of Pavia, Italy, in 2001. She is currently a Full Professor in Computer Science at the University of Piemonte Orientale, in Alessandria, Italy. Her main research interests focus on business process management, machine learning and deep learning, case based reasoning and decision support systems, with a particular interest for medical applications. She has authored more than 200 papers in international journals and international refereed conferences in Artificial Intelligence and Medical Informatics. She is a member of the Editorial Board of six international journals, Associate Editor of two international journals, and member of several technical program committees of international conferences in her research area.
Prof. Dr. Abdel-Badeeh Mohamed Salem
Ain Shams University, Egypt
Speech Title: Computational Intelligence Science In Smart Digital Healthcare Systems
Prof. Abdel-Badeeh M. Salem is a full Professor of Computer Science since1989 at Faculty of computer and information sciences, Ain Shams University, Egypt. His research includes intelligent computing, artificial intelligence, biomedical informatics, big data analytics, intelligent education and smart learning systems, information mining, knowledge engineering and Biometrics. He is Chairman of Working Group on Bio-Medical Informatics , ISfTeH, Belgium He has published around 850 papers (150 of them in Scopus). He has been involved in more than 800 International conferences and workshops as; a keynote and plenary speaker, member of Program Committees , workshop/invited session organizer , Session Chair and Tutorials.. In addition he was a member of many international societies. In addition he is a member of the Editorial Board of 100 international and national Journals. Also, He is member of many Int. Scientific Societies and associations elected member of Euro Mediterranean Academy of Arts and Sciences, Greece. Member of Alma Mater Europaea of the European Academy of Sciences and Arts, Belgrade and European Academy of Sciences and Arts, Austria
More speakers to be announced.
Previous ICIEI Dinguished Speakers
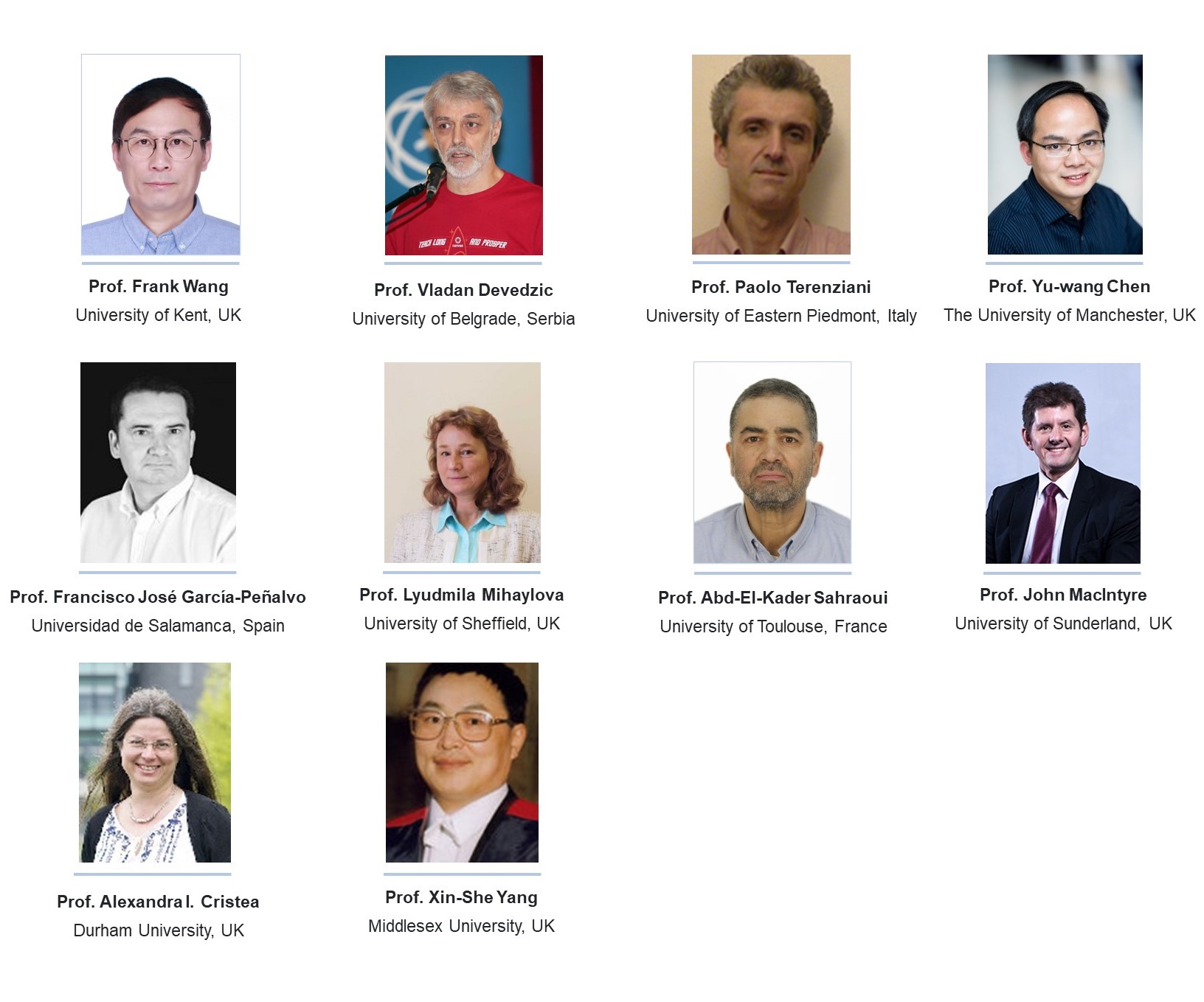